文章信息
Li, Y., Yabuki, N., Fukuda, T., & Zhang, J. (2020). A big data evaluation of urban street walkability using deep learning and environmental sensors-a case study around Osaka University Suita campus. eCAADe
论文摘要
虽然众所周知,城市街道的宜步性在促进街道质量和公众健康方面发挥着至关重要的作用,但如何定量和全面地衡量它仍没有达成共识。最近出现的深度学习和传感器网络揭示了克服先前限制的可能性,从而带来了研究范式的转变。利用这一优势,本研究探索了一条城市街道宜步性测量的新途径。在实验研究中,我们采集了覆盖大阪大学内街道的街景图片、交通流量数据和环境传感器数据,并进行了物理和感知步行能力评估。结果表明,校园街道适步性显著高于市政街道,靠近大型服务设施的街道适步性较好,而其他街道得分较低。实际步行能力和感知步行能力之间的差异表明了自动计算方法的可行性和局限性。
Abstract
Although it is widely known that the walkability of urban street plays a vital role in promoting street quality and public health, there is still no consensus on how to measure it quantitatively and comprehensively. Recent emerging deep learning and sensor network has revealed the possibility to overcome the previous limit, thus bringing forward a research paradigm shift. Taking this advantage, this study explores a new approach for urban street walkability measurement. In the experimental study, we capture Street View Picture, traffic flow data, and environmental sensor data covering streets within Osaka University and conduct both physical and perceived walkability evaluation. The result indicates that the street walkability of the campus is significantly higher than that of municipal, and the streets close to large service facilities have better walkability, while others receive lower scores. The difference between physical and perceived walkability indicates the feasibility and limitation of the auto-calculation method.
文章下载链接
http://papers.cumincad.org/cgi-bin/works/paper/ecaade2020_113
研究框架
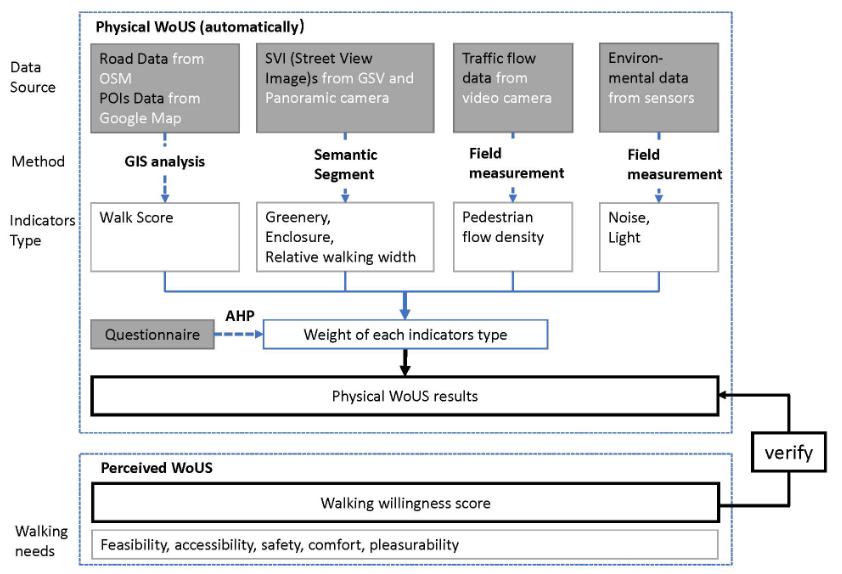