文章信息
Zhang, J., Fukuda, T., & Yabuki, N. (2021). Automatic object removal with obstructed façades completion using semantic segmentation and generative adversarial inpainting. IEEE Access, 9, 117486-117495.
论文摘要
在城市环境中完成有遮挡的立面的自动物体移除对于许多应用是至关重要的,如场景修复、环境影响评估和城市测绘。然而,以往的物体移除通常需要用户手动在不需要的物体周围创建一个遮罩,并提前获得背景立面信息,这在实施多任务项目时将是劳动密集型的。此外,准确检测城市景观中需要移除的物体,并对静态的受阻建筑立面进行涂抹以获得可信的图像,是这一目标的主要挑战。为了克服这些困难,本研究从以下两个方面来解决物体移除和外墙喷漆的问题。首先,我们提出了一种基于图像的城市景观消除方法,通过应用语义分割来检测几个类别,包括行人、骑手、植被和汽车,以及使用生成对抗网络(GANs)通过背景纹理和街道图像的修补信息来填补检测到的区域。其次,我们提出了一个工作流程,从街景图像中自动过滤未包含的建筑立面,并为基于GAN的图像涂抹模型定制了一个数据集,包括原始图像和遮罩图像。此外,引入了几个全参考图像质量评估(IQA)指标来评估生成的图像质量。验证结果证明了我们提出的方法的可行性和有效性,合成的图像在视觉上是真实的,语义上是一致的。
Abstract
Automatic object removal with obstructed façades completion in the urban environment is essential for many applications such as scene restoration, environmental impact assessment, and urban mapping. However, the previous object removal typically requires a user to manually create a mask around unwanted objects and obtain background façade information in advance, which would be labor-intensive when implementing multitasking projects. Moreover, accurately detecting objects to be removed in the cityscape and inpainting the static obstructed building façade to obtain plausible images are the main challenges for this objective. To overcome these difficulties, this study addresses the object removal with the façade inpainting problem from the following two aspects. First, we proposed an image-based cityscape elimination method for automatic object removal and façade inpainting by applying semantic segmentation to detect several classes, including pedestrians, riders, vegetation, and cars, as well as using generative adversarial networks (GANs) for filling detected regions by background textures and patching information from street-level imagery. Second, we proposed a workflow to filter unoccluded building façades from street view images automatically and tailored a dataset for the GAN-based image inpainting model with original and mask images. Furthermore, several full-reference image quality assessment (IQA) metrics are introduced to evaluate the generated image quality. Validation results demonstrated the feasibility and effectiveness of our proposed method, and the synthetic image is visually realistic and semantically consistent.
文章下载链接
https://ieeexplore.ieee.org/abstract/document/9517298
研究框架
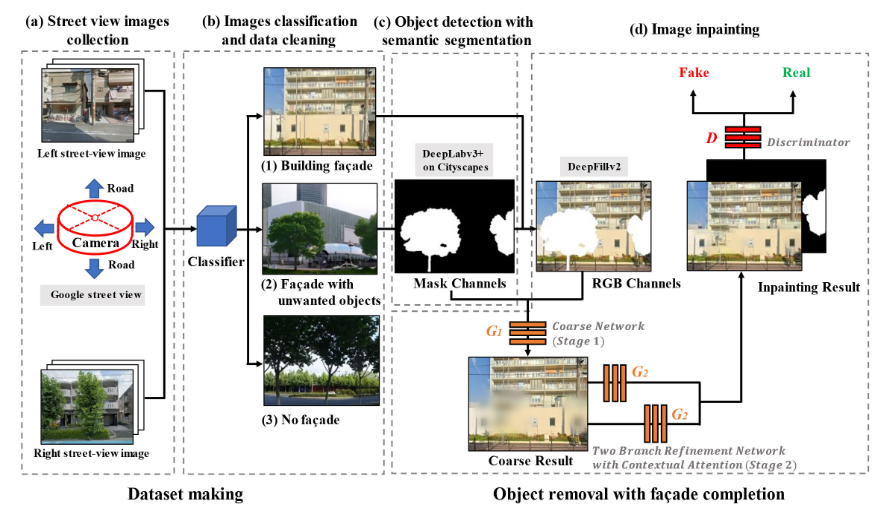
首先,我们需要建立基于GaN的图像修复的建筑立面数据集,这些图片可以从街景服务中获得,并使用分类器进行清理。然后,可以通过基于城市景观数据集的语义分割算法来检测街道上的障碍物。接着,我们介绍了一个自由形式的图像修复工具,以填补上下文关注的空白。