文章信息
Li, Y., Yabuki, N., & Fukuda, T. (2022). Exploring the association between street built environment and street vitality using deep learning methods. Sustainable Cities and Society, 79, 103656.
论文摘要
街道活力已成为评价城市街区可持续发展吸引力和潜力的重要指标,它可以通过人们在街道上步行活动的类型和频率来体现。虽然人们已经认识到街道建成环境特征会影响行人的行为和街道活力,但量化这些特征的影响仍然没有定论。本文提出了一种自动深度学习方法来定量研究街道建成环境与街道活力之间的关系。首先,通过多目标跟踪和场景分类,建立了街道活力分类的深度学习模型,实现了基于行人在街道上的数量和活动的街道活力自动评价。然后,采用语义分割的方法对选取的五个与活力相关的街道建成环境变量进行度量。接着,运用线性回归模型评价建成区环境变量对街道活力的影响。为了验证我们方法的准确性和适用性,我们选择了大阪的一个商业综合体作为例证。实验结果表明,街道宽度和透明度对街道活力有显著的正向影响。与传统方法相比,我们的方法是可行的、可靠的、可移植的、更高效的。
Abstract
Street vitality has become an essential indicator for evaluating the attractiveness and potential of the sustainable development of urban blocks, and it can be reflected by the type and the frequency of people's pedestrian activities on the street. While it is recognized that street built environment features affect pedestrian behavior and street vitality, quantifying the impact of these characteristics remains inconclusive. This paper proposes an automated deep learning approach to quantitatively explore the association between the street built environment and street vitality. First, we established a deep learning model for street vitality classification for automatic evaluation of street vitality based on the volumes and activities of pedestrians in the street through multiple object tracking and scene classification. Then, we applied semantic segmentation to measure five selected vitalityrelated street built environment variables. Finally, a linear regression model was applied to evaluate the built environment variables’ significance and effects on street vitality. To verify our method's accuracy and applicability, we selected a commercial complex in Osaka as an illustrative example. The experimental results highlight that street width and transparency have significant positive effects on street vitality. Compared with traditional methods, our approach is feasible, reliable, transferable, and more efficient.
文章下载链接

pagination_SCS_103656.pdf
研究框架
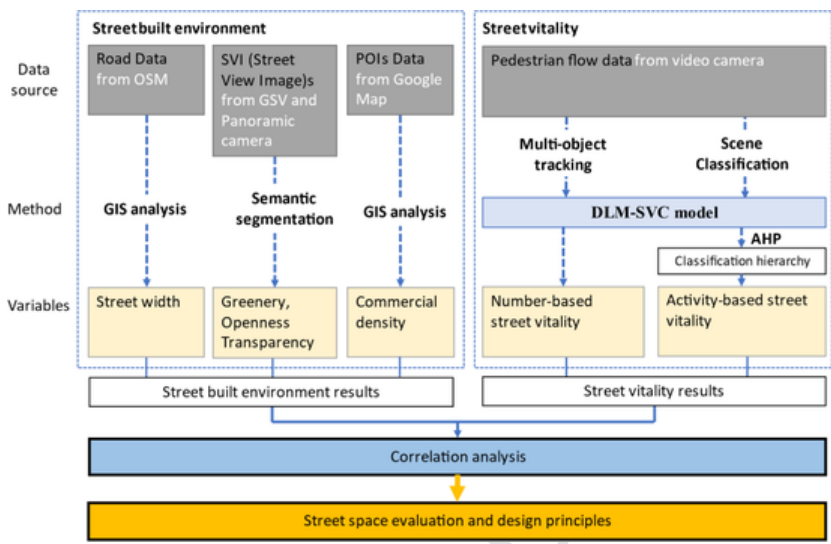
在本研究中,我们将街道活力定义为行人活动的强度,包括由行人引发和提供的活动的强度和复杂性。为了实现我们的研究目标,我们建立了一个自动量化街道活力与街道建筑环境之间关系的研究框架,包含三个主要部分。首先,我们结合GIS分析和语义分割,分析了与街道活力相关的街道建筑环境变量。其次,我们提出了一个基于行人行为强度和行人数量的街道活力分类的深度学习模型(DLM-SVC)。DLM-SVC模型包括两部分:一个使用场景分类的基于活动的模型和一个使用多个物体跟踪的基于行人数量的模型。在基于活动的模型中,使用分析层次过程(AHP)和调查问卷设计了一个分类层次结构。第三,引入线性回归模型,探索街道建筑环境变量与街道活力之间的内部关联。